How AI Can Only Work When Humans Collaborate
Machines are more intelligent than humans; is this accurate? It depends. Researchers are currently developing algorithms and machine learning (ML) models within the narrow artificial intelligence framework (NAI), which outperforms humans in most specific cases (technically called targets).
NAI candidate is a collection of ML models created and trained on historical behavioral data to learn patterns and causalities aiming to be used to solve a specific business problem. When trained and implemented in the automated pipeline, those models predict patterns and potentially impact decision-making become an AI process.
The learning
Events and objects are seen by babies and processed to create history and connectivity in their minds. Our memory stores events that allow the learning of logical connectivity between neurons. For machines, historical data replaces events and objects, which presents a substantial value of the machines given the current processing power that it can use to read and analyze years of data. On the other hand, humans need much more time to pass through the same events and objects. Moreover, a machine learning model can remember historical data patterns and make informed decisions based on what it has learned, which helps cover the needed touch points related to business processes for robust statistical learning.
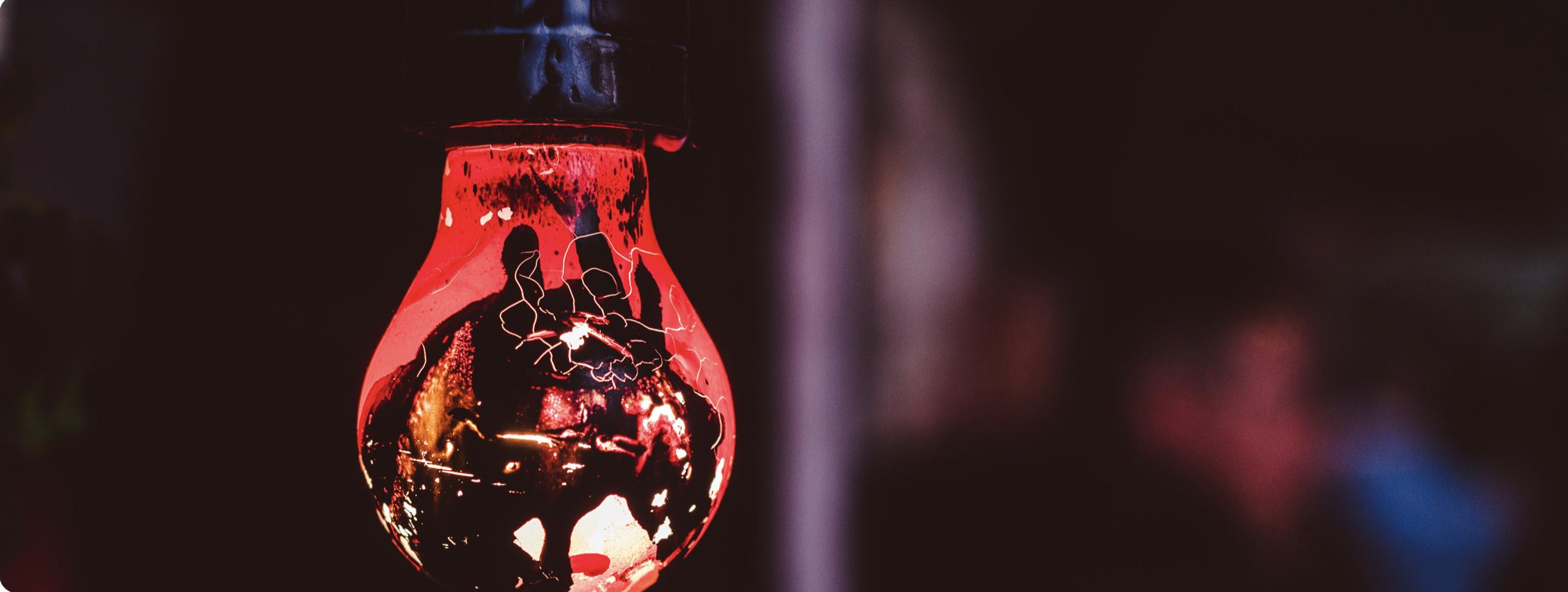
Challenges & Developments
Data availability is one of the biggest challenges for ML model training. Good data governance initiatives for CSPs have a strategic added value for the adoption of AI initiatives.
With a valid dataset, Data Scientists, with the help of subject matter experts (SMEs) in the industry specified, would develop and implement the appropriate model to solve the business problem in place, which will be later integrated by system developers for business decision making.
How can we distinguish the "appropriate model" and its characteristics? Start by defining a model; it is a mix of mathematical and statistical formulas defined by types and parameters. Data scientists define types, while parameters are learned from the data. A model becomes appropriate when solving a business problem using the right data and is implemented, given its complexity and availability.
Most of the used models fall under one of the following three types: supervised, unsupervised, and reinforcement learning. All types are designed to predict unseen events using seen/captured data. Thus, the second challenge of data scientists is to decide which model to use for each business problem.
Without zooming in into statistical and mathematical assumptions, supervised models learn and memorize the historical patterns and co-occurrence of the outcome (also called a label) and derive the optimal statistical function that links the outcome to patterns. Unsupervised is the approach of learning data variations and classifying patterns into a measurable space. For example, to develop a similar root cause of network failures, an unsupervised approach would help. In contrast, a supervised approach is a way to learn the behavior of an outcome through patterns and variations of data captured, such as predicting the type of a network failure event based on what happened before that event. When it comes to reinforcement learning, which is an innovative approach in the data science space and heavily used in certain industries, including telecom, it consists of integrating a human in model tuning and adaptation following some changes in the data flow coming to the model. Theoretically, the human is called an "agent" with a specific gain and loss utility function and trying to direct the learning towards maximizing profit.
Optimal way
The optimal model is the one with higher statistical accuracy (defined as "the appropriate model"), and minimizes training efforts and implementation/integration time with the environment generating the data. This tradeoff discussion is highly essential and should be a joint discussion between a data scientist and SMEs from the early stages of project design and roadmap. We have seen a high percentage of failed AI initiatives within organizations due to the lack of communication from the start. Data scientists tend to use the challenging mathematical and statistical approach, while CSPs are looking for a final output with clear objectives to solve a specific business problem.
B-Yond
A practical example is B-Yond's Agility products, which are based on advanced supervised learning on top of a reinforcement approach by integrating an SME (agent) in the loop for continuous feeding of relevant information from the network to the model maximize the accuracy of detecting the root cause. B-Yond's configurable black-boxes of pre-trained models are also already optimized on both dimensions: fully automated with highly intelligent models but keeping the necessary model configurations parameters available for SMEs to control models' operational aspects.
In the next series of blogs, we will go more into technical details of Agility product configurable black-boxes-AI models. We will also discuss Anomaly detection pre-trained robots that sit in CSPs environments 24/7 learning from data that report any issue and sometimes fix it automatically