Zero Tolerance For Service Interruption
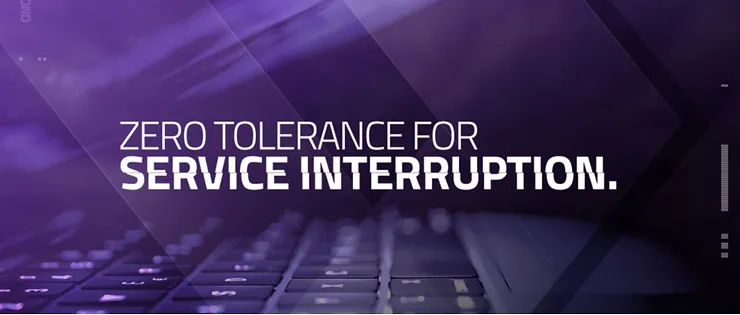
Over the last number of years, CSPs have been on a transformational journey to become more customer focused in order to remain relevant and competitive in their markets. The need for a customer-centric approach is heightened by decreasing profitability, subscriber base saturation, and competition from OTT players. This has been further compounded by the Coronavirus pandemic as working, schooling, and entertaining from home have become common. The internet connection into our homes or onto our mobile devices has transitioned rapidly from being a nice-to-have to being a necessity. All of this means that in general, but especially in this Covid-19 world, customers have zero tolerance for any service interruptions or outages.
Even after all the effort and investments made, CSPs still face a fundamental problem. Network-centric information such as performance counters and network alarms are still predominantly the main source for detecting, analyzing, and troubleshooting customer and service impacting issues.
Monitoring network-centric information to troubleshoot customer and service-centric problems leads to a couple of fundamental issues:
- In many situations, the network KPI’s say that everything is working fine, but customers are still complaining via customer care and social media channels. Even worse a percentage is churning because of their poor quality of experience.
- Network Operations Centers (NOCs) and Service Operations Centers (SOCs) are flooded with alarms. There are simply too many to effectively manage, leading to delayed reactions or completely missed incidents.
- There is no way to determine the customer impact of network incidents in realtime, resulting in investing time and effort into fixing problems that have limited or no impact on customers. In parallel, actual customer-impacting issues are going unnoticed and unresolved.
In addition, the current model of monitoring network-centric information to troubleshoot customer and service impacting issues is a break/fix model and therefore completely reactive.
Often customers have already been impacted by the time a problem is discovered. Maintaining the lead in the market is contingent on how fast a CSP can respond to their customer’s needs and meet their customers’ expectations. This is where an AI-driven detective maintenance solution that correlates data points from traditionally siloed data sources can bring tangible benefits. Leveraging AI, CSPs can transform the operation of the network from a network-centric operating paradigm to a customer and service-centric operating paradigm which puts customer and service impact at the center of every technical and business decision.
The ultimate goal of an AI-driven detective maintenance solution is to ensure that the end customers are happy with their experience of using the network and services delivered across it. If customers are happy, they will remain loyal and become a promoter of the CSP’s brand. In order to achieve this goal, it is necessary for a CSP to:
- Understand customers’ real experience and real perspective of using services, monitoring that continuously, and raising alerts when there are service quality degradations
- Correlate service degradation against network alarms, performance counters, configuration management and other relevant sources of information in order to understand where the problem is occurring
- Learn the patterns from past incidents and apply those learning to future incidents
- Understand actual and detected customer impact of network problems and when there are multiple problems, it is critical to prioritize those that truly impact or have the potential to impact customers and revenues
- Automate the root cause analysis of customer-impacting issues, using AI to provide recommendations on how those issues can be resolved and, where possible, automate the resolution to those issues back into the network before customers even realize they have an issue
- Leverage AI and ML to detect issues before they occur based on the learning from past events which enables a CSP to become truly proactive
CSPs need a solution, tailored for their specific requirements and operating environment, that can continuously monitor the end-to-end service quality and performance and raise alerts in real-time to NOC and SOC users whenever a service degradation occurs. By using re-enforcement learning, the AI/ML algorithms can learn which alerts are important and which are noise, to ensure that the NOC and SOC users only have visibility of the service degradations that are important to them and that are really impacting the customer experience.
Once a service degradation is detected, the next step is to quickly understand which customers, services, and network elements or locations are impacted. In addition, understanding the potential revenue impact allows the CSP to prioritize which service degradations should be actioned first. More importantly, AI/ML really excels at performing automated root cause analysis and determining a recommended course of action.Automated root cause analysis and determination of the recommended action require the correlation of customer and performance information with clear codes, network performance counters, network alarms, etc. in order to decipher the relationships and patterns between these traditionally disparate data sets. Once a recommended action has been determined and prioritized, remedial actions can be automated back into the network to fix problems before customers even know they have a problem.
Finally, the AI/ML algorithms embedded in the AI-driven detective maintenance solution can be used to detect future service degradations and future customer experience impacts based on historical data signatures. This enables a CSP to get ahead of the curve and become truly proactive, resolving service and customer impacting issues before they even occur or become pervasive.
The business benefits of an AI-driven detective maintenance solution are numerous. Improved customer experience means loyalty and brand equity which leads to higher retention rates and customers becoming referral ambassadors. Improved operational efficiency leads to improved service reliability and results in a CSP being able to do more with less. Finally, automation of issue resolution through the integration of recommended actions to 3rd party systems, where feasible, means faster resolution of customer and service impacting issues which ultimately leads to lower operating expenses. In these worrying times, all this will ultimately establish trust between you and your customers, dramatically increase the likelihood they will recommend you to their friends, and ensure their loyalty long into the future.